How Data Silos Act as Barriers to Generative AI Adoption in Businesses
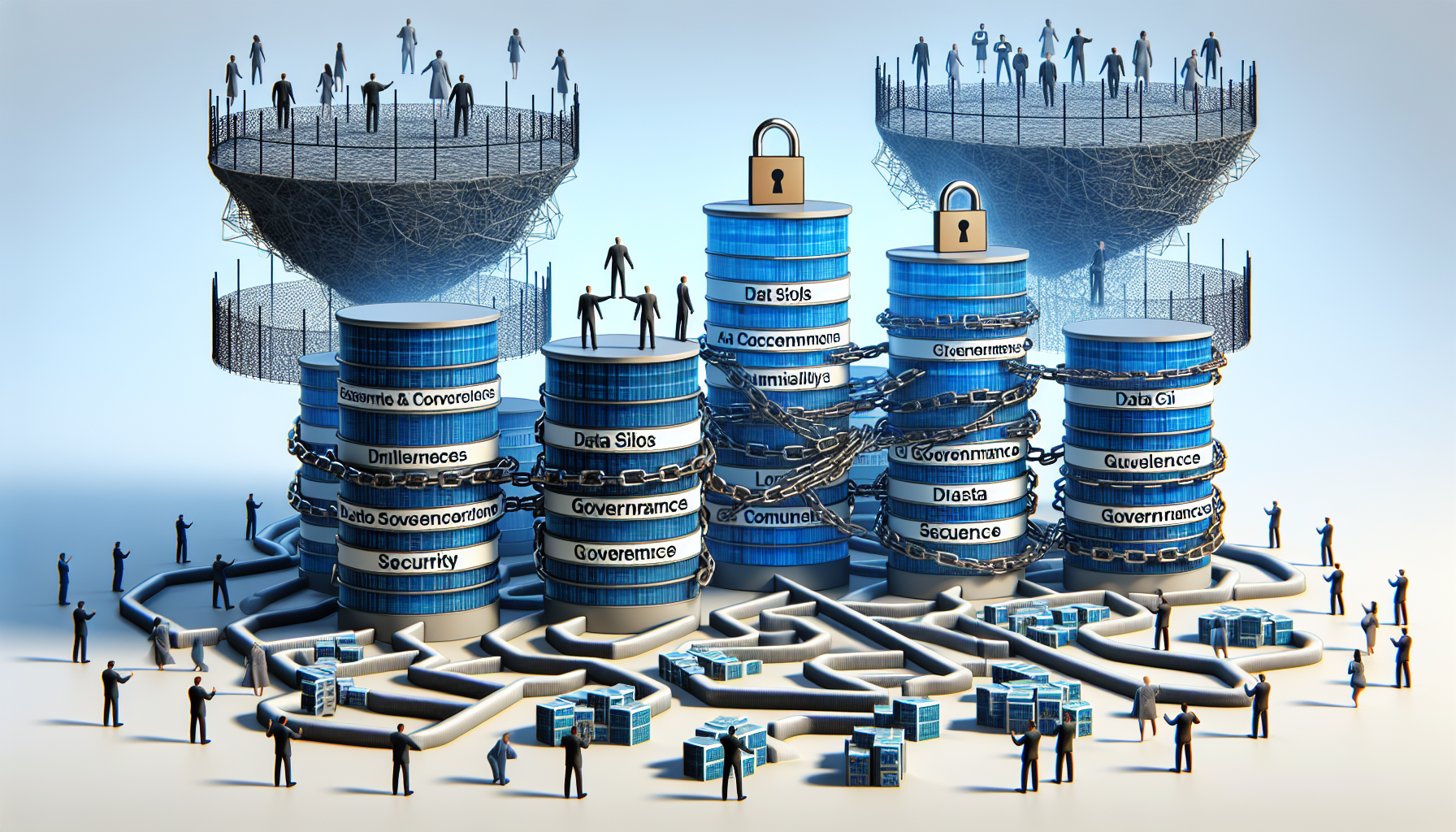
As AI adoption becomes indispensable while running a business today, a lesser-known but profoundly impactful obstacle remains- data silos. These fragmented reservoirs of information, often overlooked, are quietly sabotaging the widespread adoption and efficacy of generative AI across industries. As businesses delve deeper into the realm of AI-driven innovation, the urgent need to confront these data silos becomes increasingly apparent, with far-reaching implications for organizational success and the onus lies on business to wake up before it’s too late.
The spread of Generative AI
From predictive analytics to natural language processing, AI technologies have permeated various facets of our lives, promising to revolutionize industries and drive unprecedented growth. However, amidst this revolution lies a critical barrier: the fragmented nature of enterprise data.
Imagine a treasure trove of insights scattered across disparate datasets, like pieces of a puzzle waiting to be assembled. Some of the first use-cases within enterprises are knowledge retrieval. Instead of spending days to weeks to find some information, hidden within a document, generative AI can enable retrieval in a matter of seconds. Thus, without a sound data model, organizations cannot unlock the full potential of generative AI.
What data silos mean
Data silos refer to isolated repositories or databases of information that are inaccessible to other parts of an organization. These silos typically arise when data is stored or managed independently by different departments, teams, or systems within a company, leading to fragmentation and lack of integration across the organization.
Data within these silos often remains unshared, resulting in duplication of efforts, inconsistent information, and hindrance to collaboration and decision-making processes. In simpler terms, data silos are like separate compartments where data is stored, each compartment being inaccessible or incompatible with others. This segregation makes it difficult for organizations to have a unified view of their data, leading to inefficiencies and missed opportunities for leveraging data-driven insights.
The impact they have on businesses today
Beyond the surface-level inefficiencies, data silos wield a profound impact on every facet of business operations. Consider a retail chain trying to personalize customer experiences: fragmented customer data across online and offline channels leads to inconsistent product suggestions from AI-powered recommendation engines, reducing customer engagement and missing revenue opportunities.
Inconsistencies and inaccuracies within datasets undermine the reliability of AI-driven insights, resulting in biased recommendations, flawed predictions, and eroded trust in AI systems. This not only impedes day-to-day decision-making but also poses significant risks to long-term strategic planning and organizational growth.
The consequences of data silos extend far beyond operational inefficiencies. Take, for instance, a healthcare provider struggling with siloed patient data stored across multiple systems and departments. The fragmented data makes it challenging for AI-driven diagnostic tools to deliver accurate and timely insights, leading to delayed diagnoses, compromised patient outcomes, and increased healthcare costs.
Similarly, a manufacturing company faces challenges in optimizing production processes due to disparate data sources across supply chain operations. Without real-time visibility into inventory levels, demand forecasts, and production schedules, the AI-driven predictive maintenance system struggles to anticipate equipment failures, resulting in costly downtime and decreased productivity.
A call to change
Addressing the pervasive challenge of data silos requires a proactive approach. Organizations must invest in technologies that facilitate seamless data integration and interoperability, breaking down the barriers between disparate datasets. Additionally, fostering a culture of data-driven decision-making is paramount, empowering employees to leverage data effectively and drive innovation. Leadership plays a pivotal role in championing these initiatives, driving organizational change, and fostering a collaborative environment where data can truly thrive.
What AI-data tools can do
As the volume of data continues to grow, manually ensuring data quality and accuracy becomes increasingly challenging. AI-driven tools can help organizations overcome these challenges by automating the process of data validation, cleansing, and enrichment. These tools can analyze data in real time, identify errors or inconsistencies, and automatically correct or flag them for further investigation. This not only saves time and resources but also enhances the overall quality and accuracy of the data being used by AI applications.
How Enkrypt AI is a step in the right direction
Generative AI is set to revolutionize efficiency and productivity, but enterprises must overcome existing data management shortcomings to harness its full potential. Waiting years to clean up data and deploy AI gives competitors a chance to surge ahead. Enkrypt AI steps in to bridge this gap, offering a suite of tools that expedite generative AI adoption. Our Control Layer for Enterprise AI swiftly navigates through security, integration, and efficiency challenges, providing a swift path to leveraging generative AI's benefits. Enkrypt AI not only enhances AI visibility across the enterprise but also facilitates strategic decision-making, driving growth and innovation. By adopting our advanced AI governance solution, enterprises can leapfrog into the future, securing a competitive edge through optimized AI deployment.